Breaking: Microsoft's AI Debugger Challenges Human Programmers' Problem-Solving Skills
Technology
2025-04-11 19:19:52Content

The Debugging Dilemma in AI Coding Assistants
Despite remarkable advancements in code generation and completion, artificial intelligence coding tools continue to grapple with a critical challenge: effective debugging. While large language models (LLMs) excel at producing code snippets and occasionally suggesting repairs, they frequently struggle when confronted with complex runtime errors or intricate logical issues.
Professional developers have long relied on sophisticated interactive debugging tools like Python's pdb, which enable deep code exploration. These tools allow programmers to meticulously inspect variables, trace program execution, and comprehensively understand complex software workflows—capabilities that remain largely beyond the current reach of AI systems.
This persistent gap underscores a fundamental limitation in AI coding technologies: most large language models operate with a surface-level understanding of code, lacking the nuanced, context-aware reasoning that human developers naturally employ during troubleshooting and debugging processes.
As AI continues to evolve, bridging this debugging divide represents a crucial frontier in making artificial coding assistants truly transformative tools for software development.
Unraveling the AI Coding Conundrum: When Intelligent Assistants Hit a Debugging Wall
In the rapidly evolving landscape of artificial intelligence, coding tools have emerged as a groundbreaking technology promising to revolutionize software development. Yet, beneath the surface of seemingly miraculous code generation lies a complex challenge that continues to perplex even the most advanced AI systems: the intricate art of debugging.Intelligent Code Generation Meets Its Greatest Challenge
The Limitations of Current AI Debugging Capabilities
Modern artificial intelligence has demonstrated remarkable prowess in generating code snippets and offering preliminary solutions. However, the debugging process remains a formidable obstacle that exposes significant gaps in current AI technology. Unlike human developers who can intuitively navigate complex logical structures, AI systems struggle to provide comprehensive error resolution strategies. The fundamental challenge stems from the nuanced nature of software debugging. While large language models can produce code with impressive speed, they lack the contextual understanding and exploratory reasoning that human programmers naturally employ. Interactive debugging requires a deep comprehension of program flow, variable interactions, and subtle logical inconsistencies - capabilities that current AI tools have yet to master fully.The Human Element in Software Troubleshooting
Professional developers bring an irreplaceable dimension to debugging that AI currently cannot replicate. The ability to trace execution paths, inspect variable states, and intuitively understand complex system interactions remains a uniquely human skill. Traditional debugging tools like Python's pdb serve as extensions of a programmer's analytical capabilities, allowing for deep introspection that goes beyond surface-level code generation. This human-centric approach to problem-solving highlights a critical limitation in artificial intelligence. Machine learning models, despite their advanced algorithms, struggle to replicate the intuitive reasoning that allows human developers to diagnose and resolve complex software issues. The debugging process is not merely about identifying errors but understanding the underlying logic and potential system-wide implications.Technological Frontiers and Emerging Solutions
Researchers and technology companies are actively exploring innovative approaches to bridge the debugging gap. Advanced machine learning techniques are being developed to enhance AI's ability to understand context, trace execution paths, and provide more intelligent error resolution strategies. These efforts involve creating more sophisticated models that can learn from complex debugging scenarios and develop more nuanced problem-solving capabilities. The integration of contextual understanding, advanced pattern recognition, and deeper semantic analysis represents the next frontier in AI debugging technologies. By developing systems that can more comprehensively analyze code structure, runtime behaviors, and potential error scenarios, researchers hope to create AI tools that can approach human-level debugging capabilities.The Future of AI-Assisted Software Development
Despite current limitations, the potential for AI in software development remains immense. The ongoing evolution of large language models and machine learning techniques continues to push the boundaries of what's possible. Future iterations of AI coding tools are likely to become increasingly sophisticated, potentially transforming the way developers approach software creation and maintenance. The journey toward truly intelligent debugging systems represents a complex technological challenge that requires interdisciplinary collaboration. Computer scientists, software engineers, and AI researchers must work together to develop more advanced approaches that can bridge the current capabilities gap. As artificial intelligence continues to evolve, the dream of a comprehensive AI debugging assistant moves closer to reality.RELATED NEWS
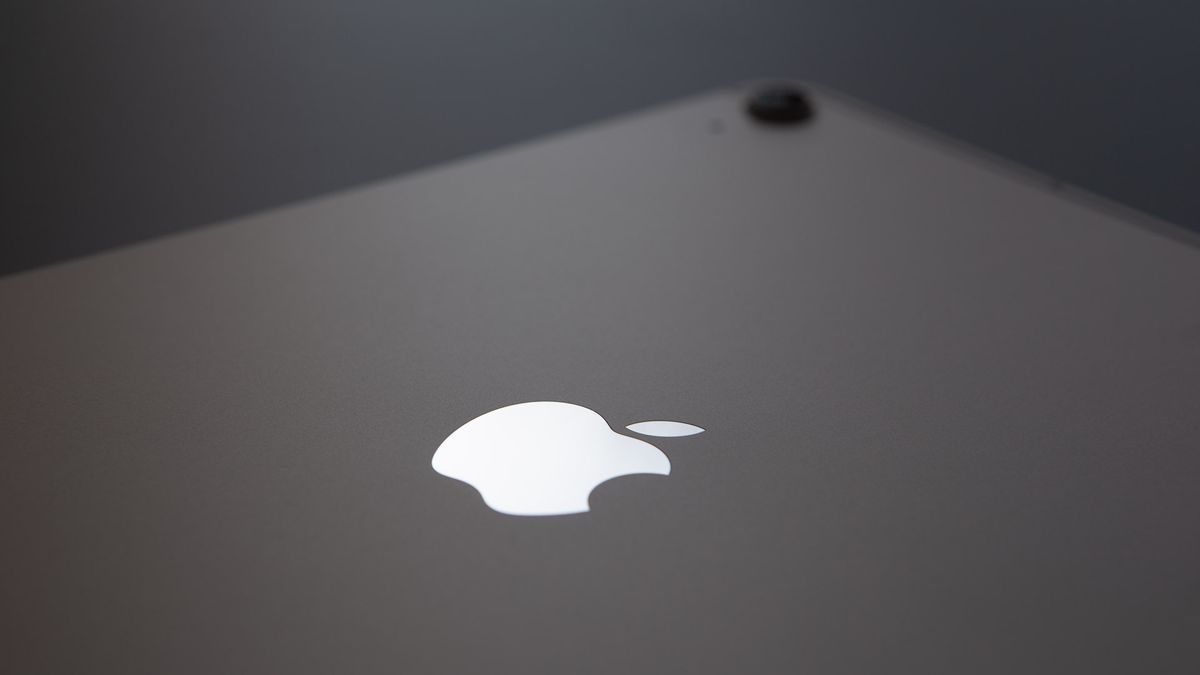
Breaking: Apple's Foldable iPad Revolution Might Be Just Around the Corner
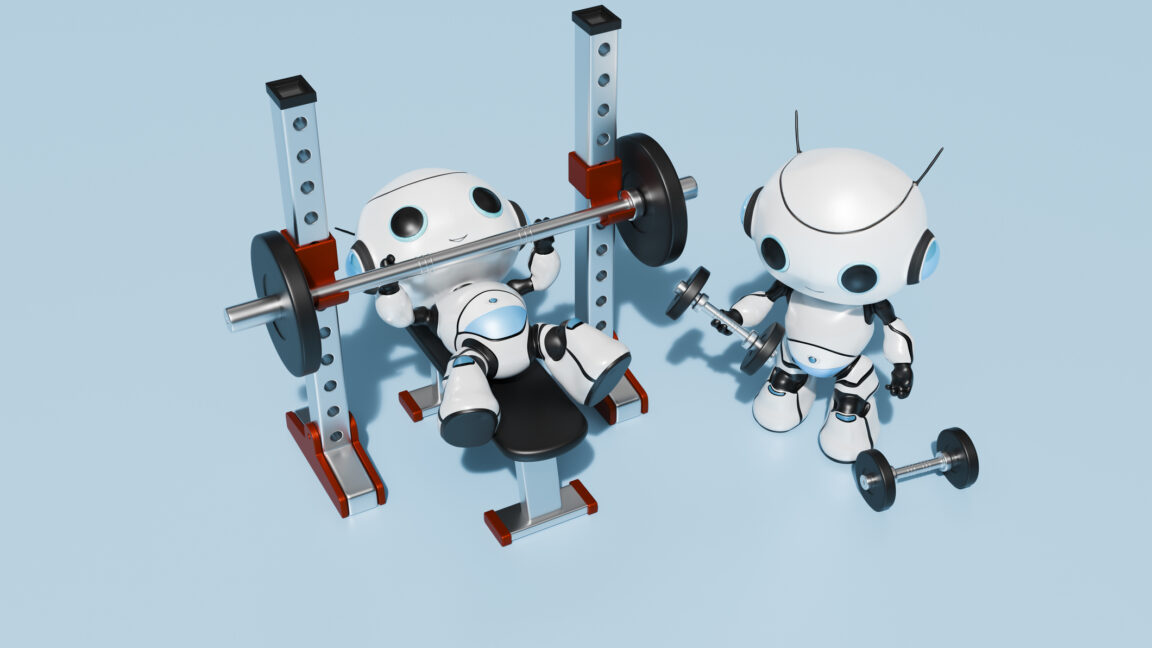